Navigating the challenges of healthcare data processing
The healthcare industry is perhaps one of the largest consumers of data in today’s digital realm. Whether it’s gaining valuable insights into patients through their clinical data or learning more about an existing drug usage through trial records, data is used in numerous ways across the industry. Although big data algorithms and analytical intelligence have been implemented, they cannot be put to their optimal use due to restrictions, system anomalies, and inaccurate results. In fact, the healthcare industry has always encountered challenges with real-time data processing and evaluation, owing to which its analytical power has been put to the test from time to time.
With this being said, healthcare organizations must realize the potential challenges and risks associated with data processing and find the most feasible and rational workarounds. Until and unless this is done, it won’t be easy to unearth valuable information from patient data or clinical records for the betterment of healthcare services, innovation of products, or research theories for new drugs. That’s why we have compiled a long list of challenges the healthcare industry is facing with data processing and management along with the feasible solutions.
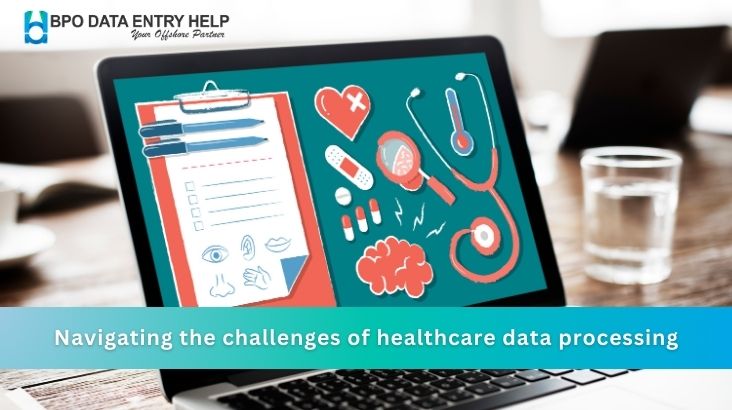
Capturing data with optimal accuracy
Firstly, healthcare data is not just limited to records presented in a structured format, like an Excel sheet showing the quarterly inventory details or the clinical trial histories. Instead, organizations need to deal with data gathered in different formats, including texts like patient surveys, images and videos like that of clinical trials and treatment processes, digital data involving electronic healthcare records, and many more. When EHR is fetched from varied sources, the compiled list of data comprises all these different formats.
Additionally, redundancy exists in the sourced healthcare data due to duplicity in records, irrelevant information, and many more. If the same data is used for AI models and machine learning algorithms, the results generated won’t be accurate and prominent. In fact, when subjected to different evaluation conditions, the data sources won’t generate consistent outputs, causing a mismatch in decision-making processes.
Fragmented patient care
Patient care is usually fragmented and inconsistent. For instance, patient vitals are usually taken regularly during hospitalization but the same can’t be said for imaging reports like ultrasound scans and X-rays. So, concluding a patient’s health condition using these diverse datasets has proven to be quite challenging. Similarly, when researchers use patient information for drug trials or vaccine studies, they need to rely on assumptions most of the time due to inconsistencies in health information and fragmented care reports.
It makes it difficult for the healthcare system to reach a proper conclusion, causing cohesion among stakeholders. Furthermore, data management for fragmented patient care cannot be done properly since the time of recording the data isn’t the same for all types. If these challenges are not addressed on time, it will be difficult to draw personalized care plans or find new drugs for the patients in the upcoming years.
Data privacy and security
Also, there have been several concerns with data security and privacy in recent times. Since most information related to patient care, drug trials, research studies, and others are confidential, they need to be encrypted and secured properly. Despite having numerous protocols implemented, maintaining optimal security and mitigating data vulnerability to zero has proven to be a huge challenge across the healthcare industry.
When electronic healthcare records are not secured optimally, they are prone to forgery and manipulation. This way, they are more vulnerable to discrepancies and mismatches in real-time processes and the collected information from historical events. The analytical results will lose authenticity and accuracy, thereby causing ethics and regulatory policies to be at stake.
Conclusion
Here, we have presented a brief review of the major challenges the healthcare industry needs to deal with data integration, processing, and management. Due to these roadblocks, enhancing the analytical ability or generating accurate predictions becomes a real hindrance. If not addressed on time, it can cause healthcare operations to come to a standstill, threatening the quality of patient care and the authenticity of healthcare reports.
Recent Post
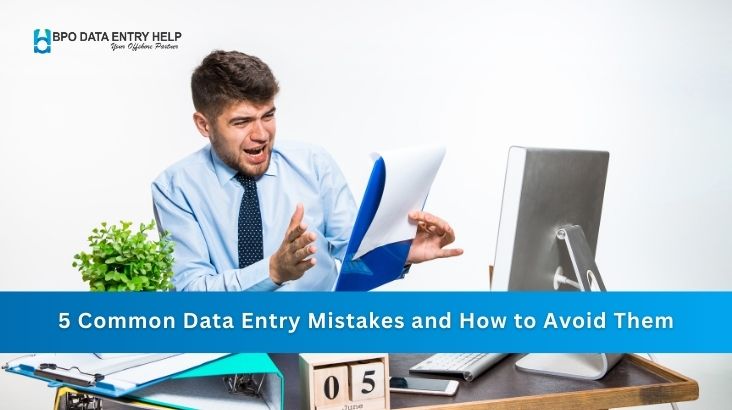
October 29, 2024
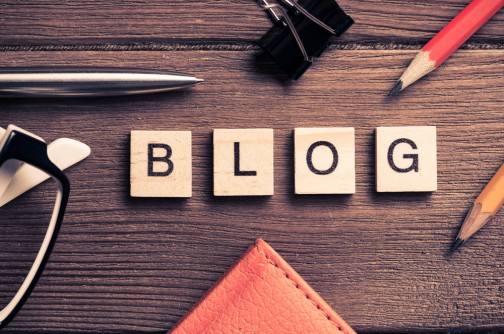
October 28, 2024
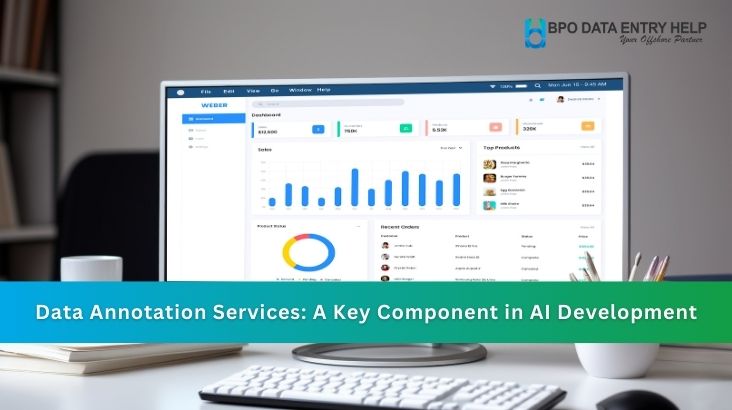
October 21, 2024
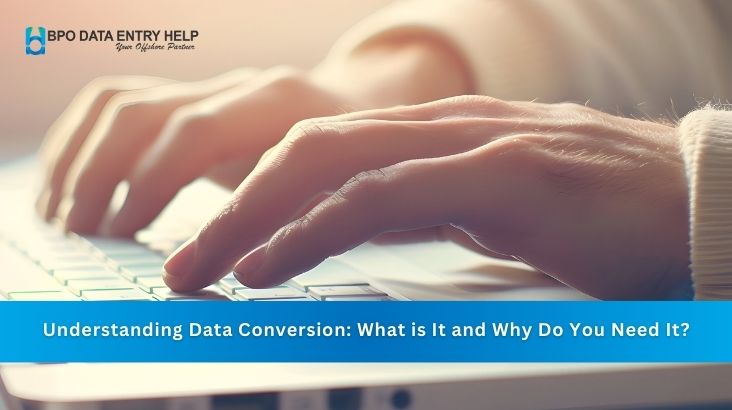
October 10, 2024
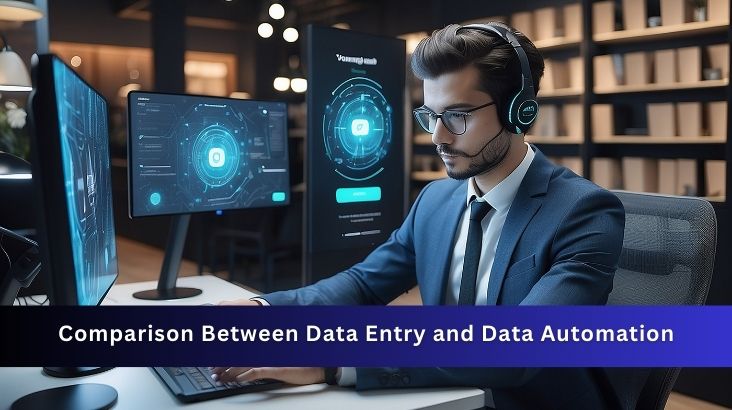
October 01, 2024